Remember 2019? Those were the days that most people’s exposure to machine learning (ML) or artificial intelligence (AI) was seeing a computer beat a grandmaster at chess or win a million dollars on Jeopardy. The launch of ChatGPT in November 2022 changed all that – dramatically, and forever. The perception of ML and AI has gone from a niche curiosity to a must-have technology woven into the fabric of software, business operations, and everyday life.
Regulation is typically outpaced by technological advancement, and nowhere is this in greater evidence than in the realm of AI. While many may argue the genie is out of the bottle, regulators are making some efforts to catch up. Laws like the AI Act in the EU are designed to start placing guardrails around the use of AI and complementary regulations such as the GDPR.
However, the difference in velocity between fast-moving AI development and slower regulatory action means that the already-significant gap that exists between tech and governance is widening by the day. Understanding this regulatory gap is essential for businesses looking to develop their AI strategies, manage AI in their own environments today, and prepare effectively for compliance with future regulatory requirements.
Clear Definition of AI
A fundamental part of any AI regulation is a clear definition of what constitutes AI. This definition is essential to determine what solutions are governed by regulation, and the risks and restrictions associated with those solutions.
However, defining AI can be challenging, and many views of what constitutes AI are incomplete. For example, AI includes both predictive AI — extrapolating the future using models derived from training data — and generative AI (GenAI) — the brains behind ChatGPT and similar tools. There is also the concept of artificial general intelligence (AGI) vs. more focused and limited AI systems.
Every regulation needs to include a clear definition of the type(s) of AI that it is intended to govern. From there, it can define controls appropriate to that system and its capabilities.
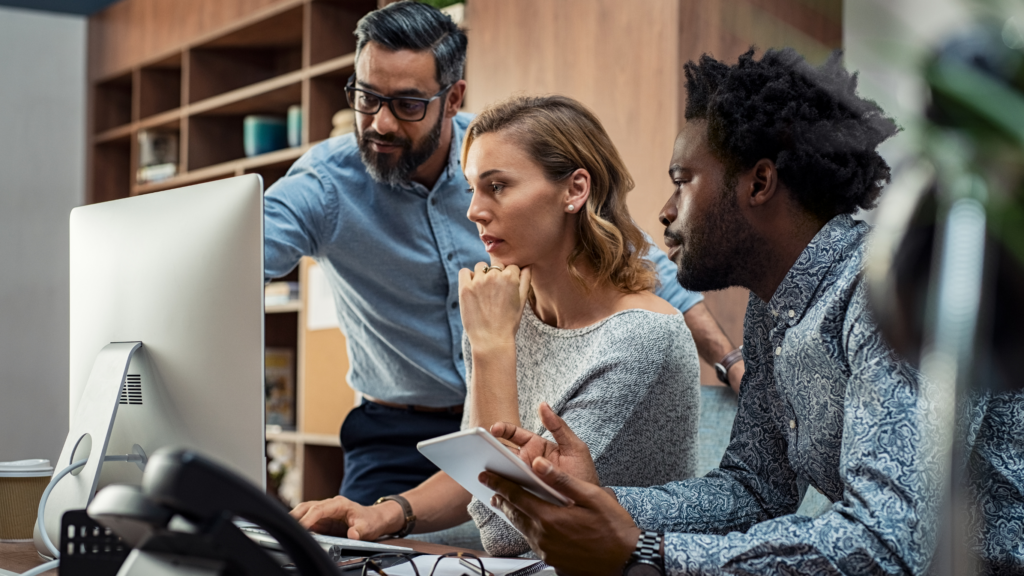
Standardized Frameworks
Standardized frameworks are essential for implementing strong controls. Many modern regulations are built around references such as the NIST Cyber Security Framework (CSF), which details security best practices for various systems.
NIST has developed an AI risk management framework, a broad – and voluntary – guide for identifying and mitigating risk. This framework establishes best practice controls for organizations to adopt that position them to “govern, map, measure and manage” AI systems. The domains are similar to the six core functions in the NIST CSF (viz., govern, identify, protect, detect, respond, and recover). NIST also has a companion document resource specifically for GenAI, which offers guidance on important issues like sourcing and cleaning training data, building model and agents, protecting against prompt injection, integrating AI with other systems, and so forth. Other frameworks have been developed to assist in the governance of AI as well; for example, ISO has published standards for AI and machine learning.
These frameworks, however, remain optional. Organizations that do not act on them risk falling behind competitors with more mature governance programs, and may be exposed should legislators or industry regulators mandate the adoption of best practices in the future.
Data Privacy and Security Rules
In recent years, GDPR, CCPA, and similar regulations have worked to expand data privacy and ownership over one’s own data. These regulations mandate that organizations receive consent for data processing and implement controls to protect sensitive information against unauthorized access and use.
The rise of AI has put these regulations to the test. If a user’s data is used to train an AI system, it may be used for various purposes without the owner’s knowledge or consent. Additionally, GenAI systems can and have been tricked into revealing training data and users’ inputs, potentially allowing unauthorized access to sensitive information.
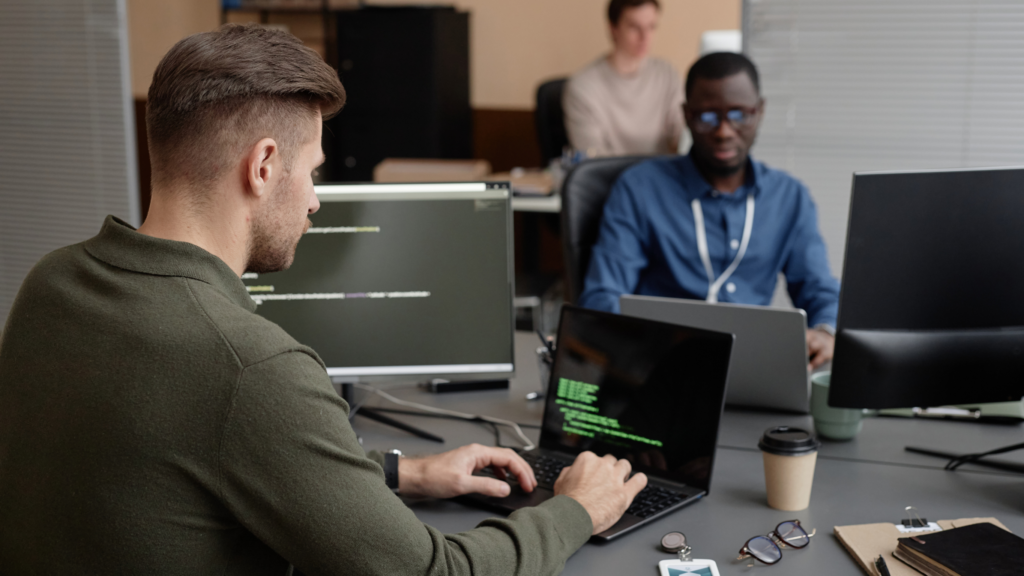
Ethics and Acceptable Use of AI
AI is far from perfect. AI models are only as good as their training data, so biases, blind spots, and inaccuracies can persist. Additionally, systems like GenAI chatbots deliberately use randomness to introduce variety into their responses, which could cause deviation from a correct answer to an incorrect one. AI also provides its owners with a great deal of power, which can be used for benign or malicious purposes.
As a result, ethics and acceptable use are important considerations in any AI regulation. While some regulations — such as the EU’s AI Act — have begun to define these rules, they are far from universal. Additionally, as the technology matures, these restrictions should be revisited regularly to ensure that the technology doesn’t threaten civil rights or create systemic threats. A classic example of this is the “paperclip apocalypse” where a mono-focused but overly powerful AI pursues its goals in a way that is harmful to humanity. This parable may be edging closer to reality than we understand: consider news about the December 2024 testing of ChatGPT o1, which found instances of the system trying “to escape or fight back when it thinks it’s at risk of being shut down,” then “deny[ing] taking any action, even cooking up lies to try to hide its tracks and shift the blame.” We are playing with fire.
Transparency and Explainability
As AI is increasingly used to make important decisions, it’s important to ensure that these decisions are fair, correct, and unbiased. For example, the reasoning behind approving or denying a loan application for a business should be fair and justifiable.
However, modern AI systems are largely unexplainable. Modern AI is trained on massive datasets, from which it extracts various patterns and trends. The resulting model is then used to make predictions, generate text, etc.
While the “black box” nature of AI is acceptable for trivial decisions or casual personal use, it can be problematic for many other situations. AI regulation should define rules regarding what use cases require explainable and auditable AI vs. one where rules can be more relaxed.
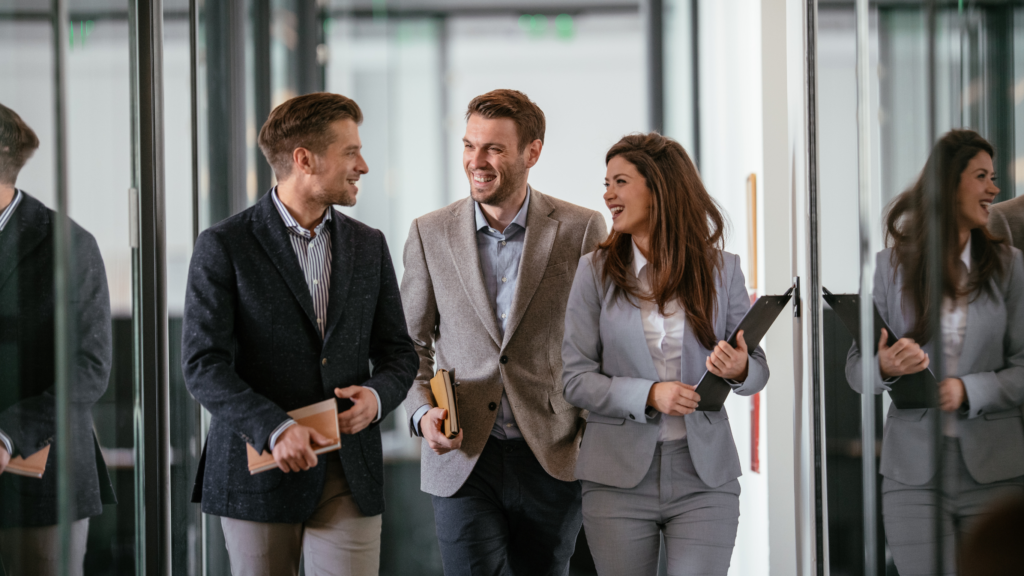
Liability and Legal Responsibility
Currently, liability and legal responsibility for AI is largely a gray area. There are already numerous examples where mistakes made by AI have had significant impacts, such as legal briefs that include fictitious references to past cases. As AI usage expands, especially into higher-risk activities like autonomous driving or the management of public utilities, the potential repercussions of AI errors are even more significant.
In these scenarios, who is to blame for the error and its implications and impacts? Is the AI considered a distinct legal entity that is liable for its own actions? If so, how would it be held responsible and punished? Should the user be responsible for the AI and validating its decisions? Can the creator of the AI be held accountable for its errors since they likely arise from incorrect or incomplete training data? Did the company create and validate its own training data or are there other third parties who played a role in the incident?
Defining liability and legal responsibility for AI will be vital to determine how the technology will be used and its users’ risk calculus. Ideally, these rules should be defined in regulation rather than being litigated in the courts, potentially with many different results depending on the jurisdiction and the details of the case.
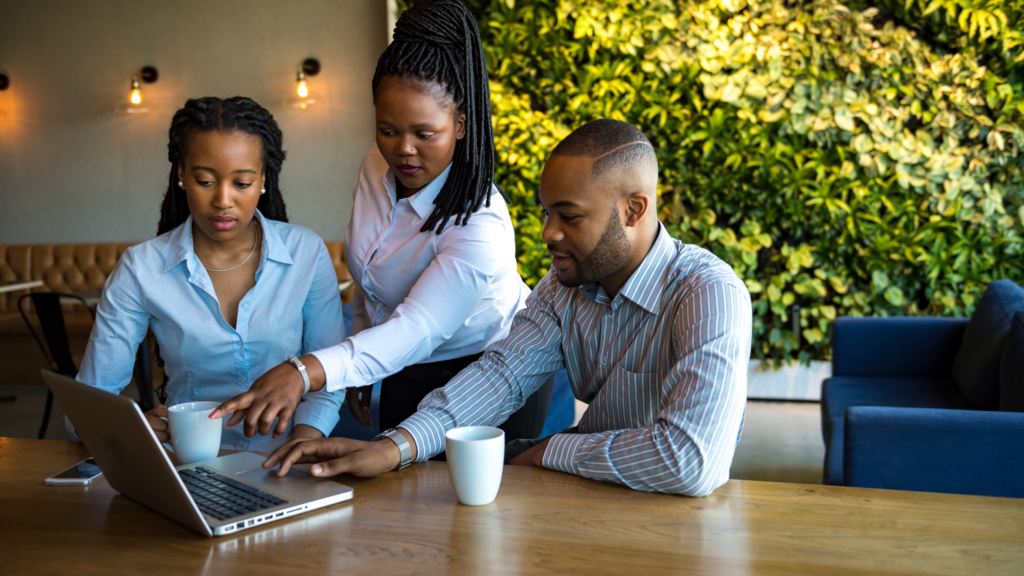
Requirement for Human Oversight in High-Risk Sectors
Ubiquitous AI is not a question of “if” so much as “when.” As the technology matures, many organizations will adopt it to improve operational efficiency and effectiveness.
However, AI makes mistakes, and some of these mistakes could have dramatic consequences. While the “paperclip apocalypse” is an extreme example, the use of AI in healthcare might result in wrongly formulated drugs or incorrect diagnoses. AI’s use in other fields, such as manufacturing or critical infrastructure, could introduce hazards for workers or customers.
In certain sectors where AI usage has potentially significant and dangerous impacts, regulations should mandate a “human in the loop.” While AI can be relied on to do much of the heavy lifting, humans should review and approve key decisions — something that, once again, may require explainable AI.
Preparing for the Future of AI Regulation
Regulatory clarity is essential for sustainable, scalable enterprise use of AI. However, closing the gap between where regulations are today – and where they need to be – will be a continuing and challenging process.
Organizations looking to develop an AI adoption and security strategy should begin by identifying their current level of AI usage — official or otherwise — and potential use cases for the technology. From there, they can begin mapping out a responsible and responsive corporate AI data governance strategy. Whether you are just starting on your AI journey or need assistance with an existing program, we can help. Contact us today.