AI is the buzzword on everyone’s lips – and for good reason. Artificial intelligence is fast emerging as a part of day-to-day life, changing the way we play, learn, and work. However, governance strategies for protecting the data that is the lifeblood of AI systems have not always kept pace with tech advancements.
Effective AI data governance frameworks not only safeguard data privacy but also mitigate the risks associated with data breaches and misuse. They support the ethical deployment of AI by addressing issues such as bias prevention and content reliability. And, crucially, by protecting the quality and integrity of your data, you help maximize your return on investment in transformative AI technologies. By establishing clear policies and controls, organizations can navigate the complexities of AI regulation and confidently use AI as a business differentiator.
Understanding the Risks
AI is a powerful technology that creates enormous opportunities, but carries a wide variety of potential risks. These risks can vary based on organization, use case, and so forth; however, some of the most common include:
- Sensitive Data Exposure: The use of unsanctioned generative AI (GenAI) tools can result in sensitive corporate information being leaked. A cautionary example of this risk was the Samsung incident in 2023, wherein sensitive corporate data was entered into ChatGPT, making it available for training the public model and to other users. An AI data governance strategy and AI policy structure can help educate users and manage this risk by controlling use of GenAI without banning it entirely (which was Samsung’s initial response in the wake of the incident).
- Regulatory Non-Compliance: Current regulations such as GDPR have rules regarding the use of AI systems. Other legislation is in now in effect (e.g., the EU’s AI Act) or in the works (e.g., Canada’s Artificial Intelligence and Data Act). A failure to manage how data is used in AI systems could result in regulatory penalties or legal action. Monitoring the fast-changing compliance landscape is a must.
- Unreliable Results: GenAI systems incorporate an element of randomness, which can contribute to the appearance of creativity but also means that they might not produce consistent outcomes. In one classic example, a car dealer’s GenAI-powered chatbot offered to sell a car to a customer for $1 (though the offer was later retracted). Without human oversight, these errors could cause financial losses, reputational damage, and other potentially disastrous impacts for the business. This so-called creativity is behind the phenomenon of AI “hallucinations” – when the LLM cannot find a valid answer to a question, it will construct a response that seems real, but can be complete fiction. Remember that current AI models do not have the capacity to “understand” or stick to the logical paths and guardrails that seem intuitively obvious.
- Biased AI Models: The large language models (LLMs) used by AI are trained on data, and the quality of the training data affects the usefulness of the developed model – positively or negatively. For example, one frequently-cited paper describing a machine learning algorithm for identifying skin lesions was later found to have a higher probability of detecting cancer if a ruler was in the image. Dermatologists include rulers to measure lesions of particular concern, so the algorithm had an unintentional bias when it was being trained. training to detect these issues. It is critical to understand the far-reaching impacts of bias in AI data management.
Implementing an AI data governance strategy provides an organization with a level of protection against all of these potential threats. AI-specific risk management standards such as the NIST AI RMF and ISO/IEC 42001 are good starting places for developing strategies that can be adapted and right-sized to your organization.
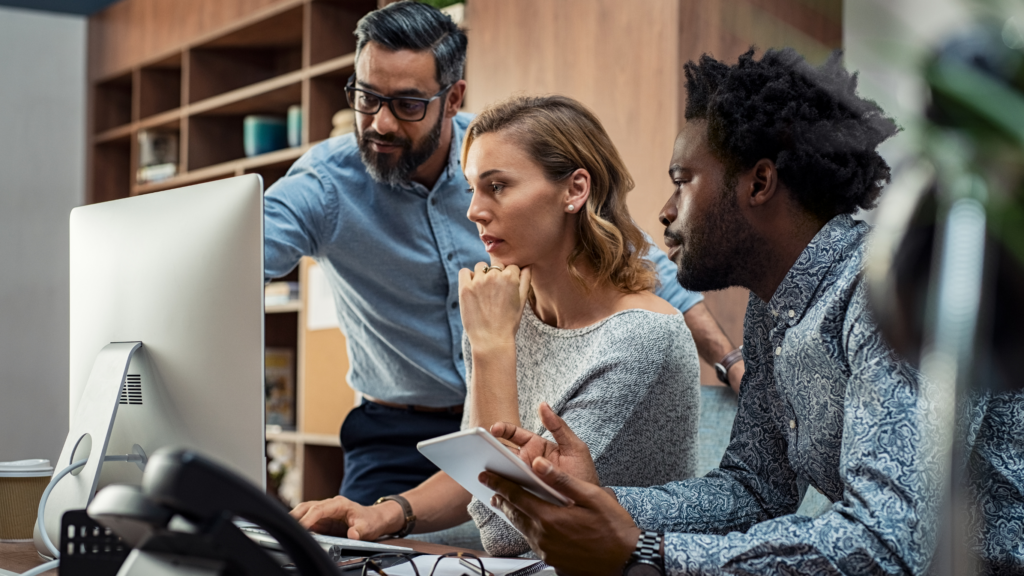
Training Data: Key Questions to Consider
An AI system is only as good as its training data. AI models are trained on large volumes of data designed to teach them important patterns in the data. These patterns allow some AI systems to classify data, while others – such as large language models (LLMs) – use their models to produce text in response to queries.
However, ensuring good quality training data poses a significant challenge for an organization. Some key considerations include data quality and data security.
1. Data Quality and Usability
An AI model is most effective if it is trained on the “right” data. Some key questions to ask include:
- Is the data reliable? Training data is used to teach the AI about important patterns. If the data is unreliable, so is the model that it’s being used to train. Poor quality data, biased content (intentional or not; malicious or not) can poison your LLM.
- Does the company own the data? Some data in a company’s possession may not be appropriate for training AI. For example, some regulations restrict the use of personally identifiable information (PII) to train AI systems without the data subject’s consent.
- What data isn’t being collected? A training dataset should include as much information as possible to maximize the accuracy of the AI model. If important data is missing from the training dataset, the model will be flawed.
- What data shouldn’t be collected? More data isn’t necessarily better. Including unreliable, low-quality, or repetitive data could skew the AI model or unnecessarily increase training time.
- How is the data being validated? Even data from a reliable source may be flawed, corrupted, or incomplete. Data validation is essential to ensure that collected data is correct and complete.
- How can the data legally and ethically be used? Regulators have begun restricting the potential uses of AI systems. It is imperative that you consider the ethics and appropriateness of using various types of data – especially PII. Depending on the use case, data may need to be anonymized, masked, or tokenized. This best positions you to mitigate privacy risks while getting the greatest value out of the data for LLM training purposes.
- How does data usage affect corporate policies? Your use of certain types of data may require you to change the way you seek customer consent, or trigger updates to your data privacy and usage policies, both internally and externally. Addressing these issues in advance will help avoid embarrassing and costly consequences down the road.
2. Data Security Considerations
Datasets used to train AI models can contain highly sensitive data. Additionally, knowledge of training data may enable an attacker to develop attacks that take advantage of loopholes or flaws in the training data. Some important questions to ask regarding the security of training data include the following:
- How is data being collected and used? Understanding data sources and collection mechanisms is essential to ensuring data security. Insecure collection mechanisms could allow an attacker to eavesdrop on data or manipulate it. Proprietary data may be suitable for training an internal LLM, but not appropriate to be shared externally.
- Where is the data stored? An organization’s decisions on how to store training data can also impact its security. For example, cloud storage may be tempting due to easier scalability and reduced costs; however, misconfigured cloud storage could leave data publicly accessible.
- Who should (and shouldn’t) have access to data? Controlling access to AI training data is essential for data security, model accuracy, and regulatory compliance. Access controls should be defined based on the principle of least privilege, minimizing access based on need to know and logged appropriately.
- How is data protected against leakage? AI training datasets are usually a large pool of very valuable information. They must be protected against theft using a defense-in-depth strategy incorporating access controls, encryption, and data loss prevention.
- How is data protected against modification? Data poisoning attacks could introduce corrupted data into training datasets, compromising the accuracy of the resulting model. Encryption, access controls, and digital signatures are examples of security controls that can be used to protect the integrity of training data. Rollback capabilities should be incorporated to enable recovery from data corruption.
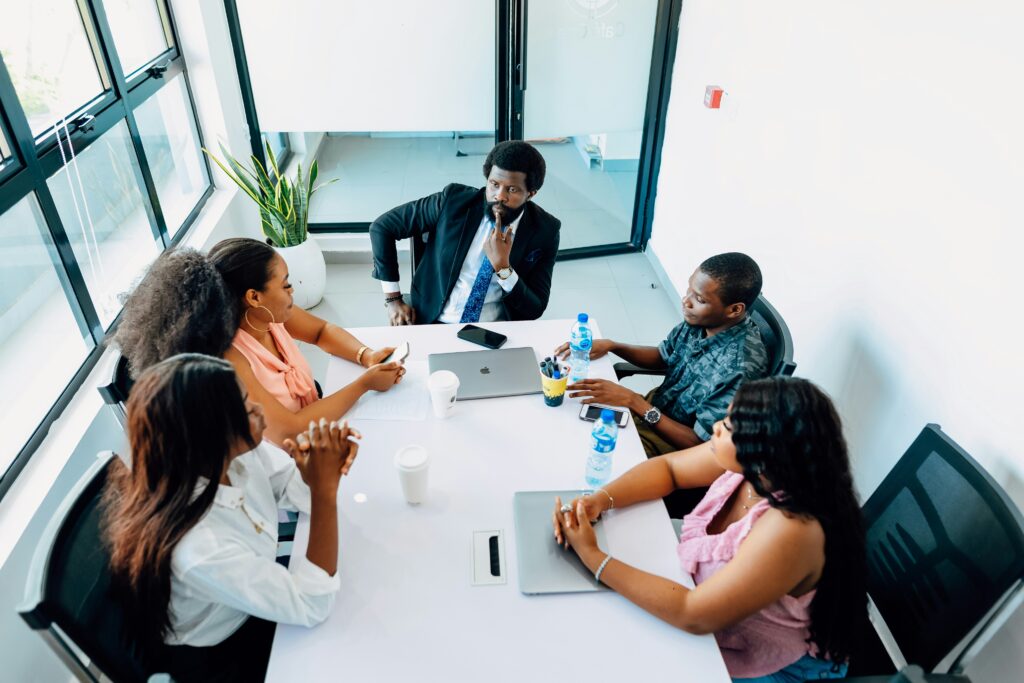
Creating Your AI Data Governance Strategy
Over time, AI will be inextricably linked to nearly every aspect of business operations. How will you maintain compliance, protect sensitive data, and build trust in your models? Having an AI data governance strategy in place in advance can help you manage this tech revolution and contain the potential cybersecurity threats, reputational damage, and the risks associated with poor AI training data.
Designing and implementing such a strategy requires a deep understanding of how AI works, and a recognition of the exciting opportunities – and potential threats – that come with AI. Learn more about the security implications of AI or reach out to ISA Cybersecurity for guidance in developing your AI data governance strategy.