As Artificial Intelligence (AI) becomes a key aspect of how most organizations operate, Canadian companies need to pay close attention to how AI and data management work together. While appropriate governance is essential, it may be hard for organizations to see how to get started on a cohesive plan. As experts in the field of AI implementation and data management, we have seen firsthand the transformative power of a well-crafted AI Data Governance Strategy – and the consequences of failing to plan ahead. A strategic approach not only ensures compliance and mitigates risks but also helps you realize the full potential of AI-driven insights while maintaining ethical standards.
In this article, we’ll explore the eight essential steps necessary to build a comprehensive AI Data Governance Strategy, addressing key considerations such as data quality, privacy, transparency, and accountability.
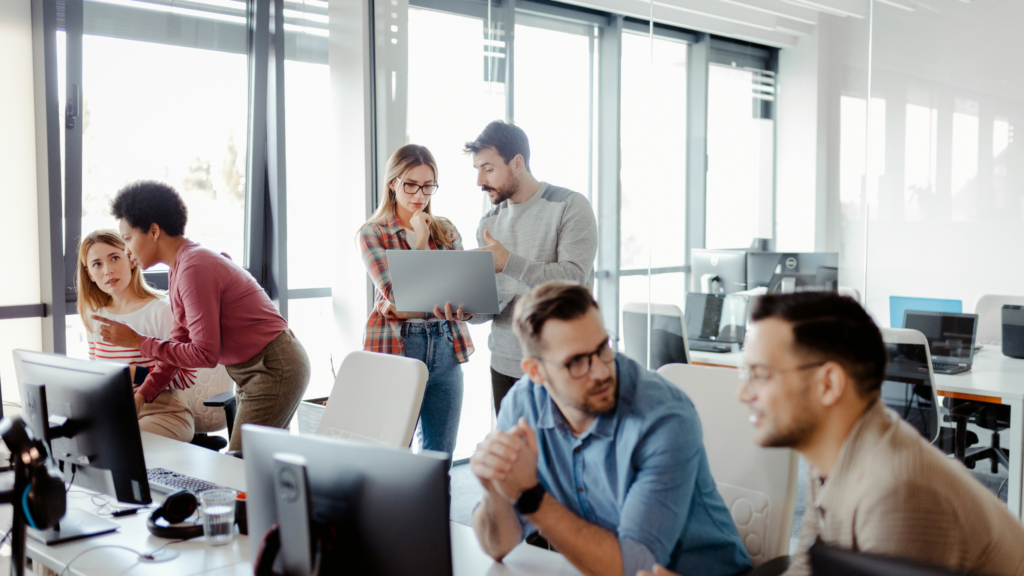
Step 1: Establish an AI Governance Framework
Choosing the right governance framework and control objectives (COs) is a crucial starting point for your project. Right-sizing your model will help you control the scope of your initiative, and make the resulting strategy more useful and relevant to your business. This will involve two phases:
Leadership and Oversight
Form an AI governance committee with representatives from legal, IT, security, data science, HR, and relevant business units. Ensure your team is enthusiastic about the initiative, and will have the time to contribute meaningfully and in a timely fashion. Some key activities for your committee:
- Define roles and responsibilities for AI governance within the organization
- Establish clear accountability structures and a RACI for decision-making
- Conduct a needs assessment and develop a roadmap
- Use the rest of this article as a playbook to help guide the process
Policy Development
Start to work on a set of comprehensive AI policies covering ethical use, data protection, and compliance. This can be complex, but fortunately there are several resources you can leverage as precedents. Consider:
- IEEE’s AI Policy Committee (AIPC);
- NIST’s AI Risk Management Framework;
- ISACA resources;
- World Economic Forum (WEF) publications; and
- Supplemental resources from Responsible AI, com, and eWeek
Using these resources, you can develop your own guidelines for AI model development, deployment, and monitoring, and establish protocols for handling sensitive data in AI systems (e.g., what to store, who can access it, anonymization techniques, etc.)
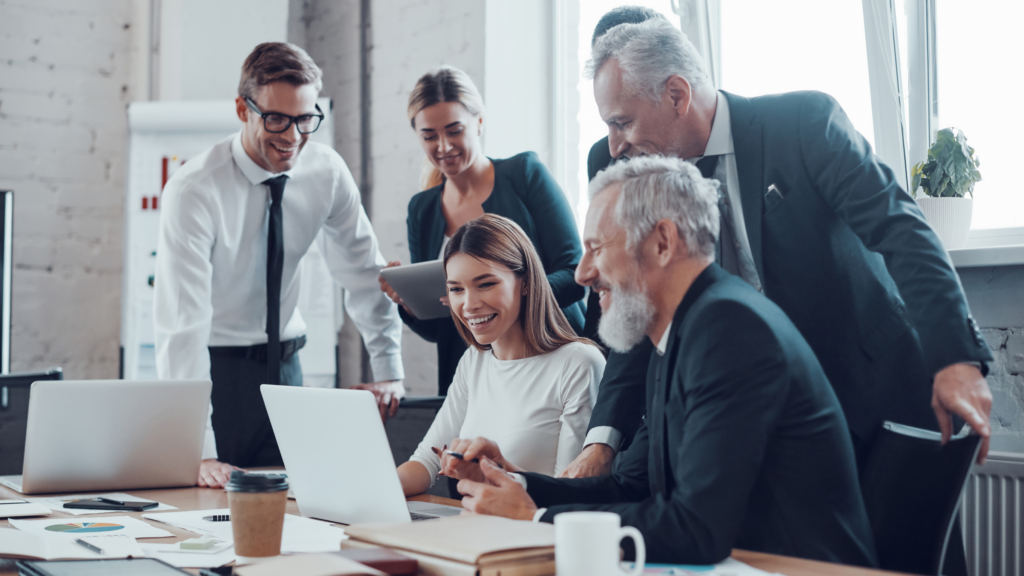
Step 2: Data Governance and Management
The second phase of the project gets into more detail. As discussed in our article on AI data governance fundamentals, there are several important decisions that need to be made regarding data quality, security, and privacy:
Data Quality and Integrity
- Implement data quality management processes.
- Establish data validation and cleansing procedures.
- Develop metadata management practices.
- Establish procedures for testing and validating your data, looking for biases or other unintended results.
Data Security and Privacy
- Implement robust data access controls and encryption.
- Ensure compliance with relevant data protection regulations – many of the same organizations that you leveraged for building your AI policies offer resources on the evolving compliance requirements in your jurisdiction or industry sector.
Data Lifecycle Management
- Define processes for data collection, storage, use, and disposal – in some cases, these processes will mirror the consents you’ve secured for the use of customer data to feed your LLM.
- Establish data cataloging and classification systems.
- Establish data retention and deletion policies.
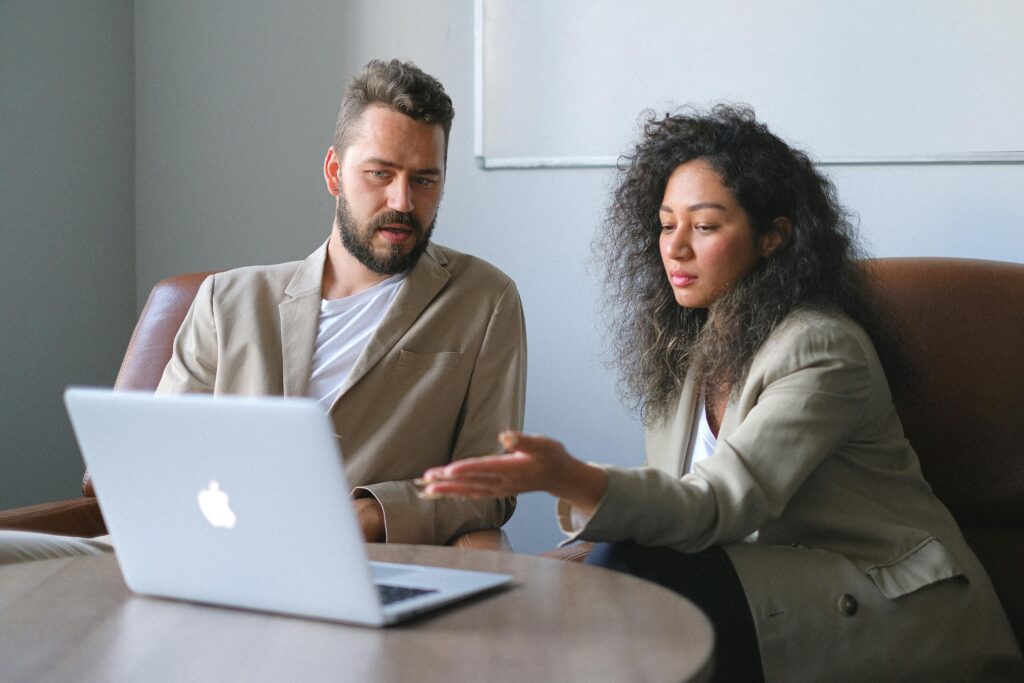
Step 3: AI Model Governance
The core of your AI system is your chosen LLM (large language model). In simplest terms, your model is essentially a complex algorithm that has been trained on a set of data to recognize certain patterns to provide responses based on your queries. Having an evaluation process to select models is an important aspect of governing your implementation – particularly as your organization becomes more and more reliant on AI. Some of the key areas to consider:
Model Development
- Establish guidelines for model selection and fine tuning.
- Implement version control for AI models.
- Define processes for model testing and validation.
Model Deployment
- Create protocols for model deployment and integration.
- Establish monitoring systems for model performance.
- Develop procedures for model updates and maintenance.
Explainability and Transparency
- Implement methods for AI model explainability.
- Establish processes for documenting model decisions.
- Develop protocols for addressing algorithmic bias.
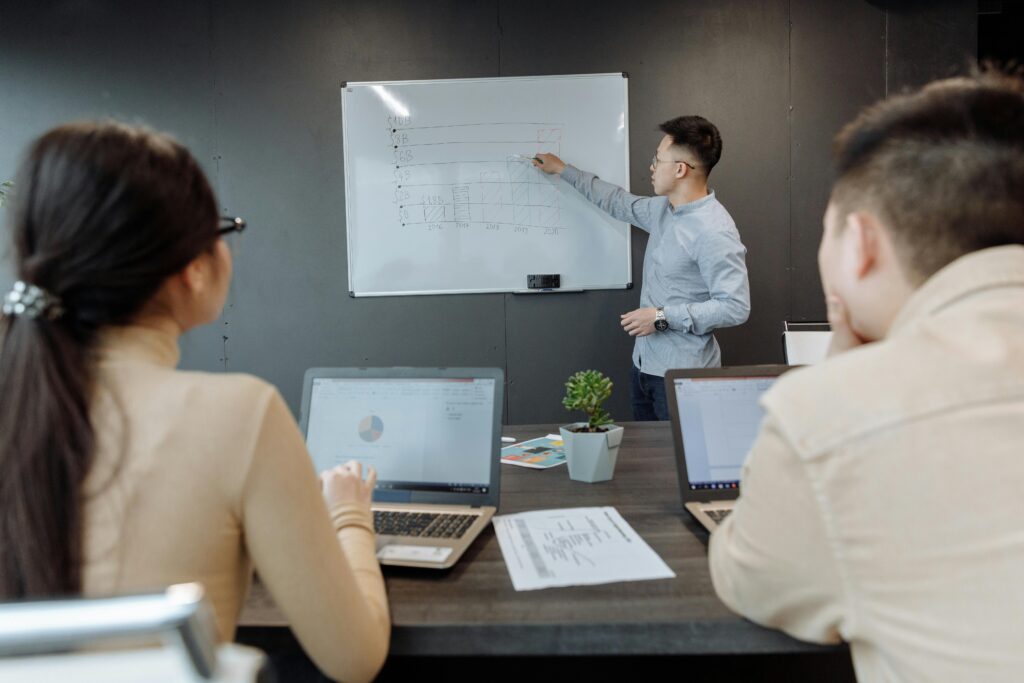
Step 4: Risk Management & Compliance
As with most aspects of the business, risk management and compliance issues must be understood in order to help you identify, assess, and mitigate potential risks, and ensure adherence to current (and emerging!) laws, regulations, and ethical standards. The key elements of this step will be familiar to most executives, and they fully apply to AI deployments:
Risk Assessments
- Conduct regular AI risk assessments and a risk matrix specific to AI applications.
- Establish risk mitigation strategies.
- As an output, ensure your organization updates its incident response and communications strategy to prepare for issues surrounding the use of AI.
Compliance
- Develop a Control Objective Framework (COF) to govern your AI program implementation.
- Ensure adherence to current relevant AI regulations and standards.
- Implement processes for ongoing compliance monitoring internally, and as the landscape evolves.
- Establish procedures for addressing non-compliance issues.
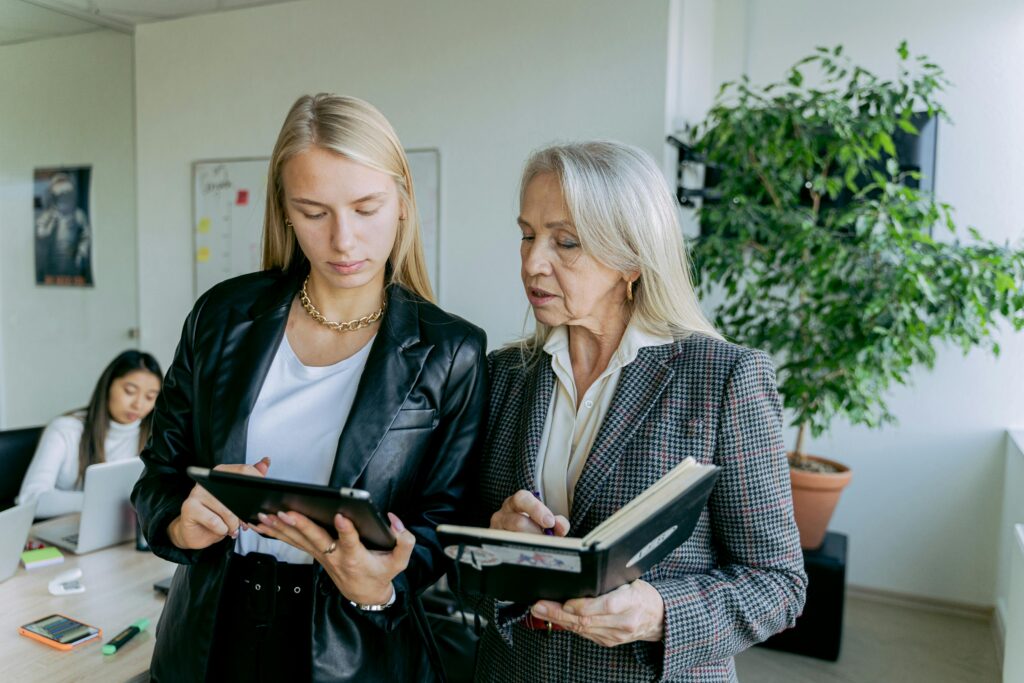
Step 5. Appropriate AI Use
In the rush to use AI, crucial considerations like ethics and bias are often forgotten. The genie may be out of the bottle when it comes to AI, but this is no reason – or excuse – for you to ignore this in your planning and deployment.
Ethical Guidelines
- Develop ethical principles for AI development and use.
- Establish an ethics review process for AI projects.
- Create guidelines for responsible AI innovation.
Bias Mitigation
- Implement processes for identifying and mitigating AI bias.
- Establish diverse teams for AI development and testing.
- Develop protocols for ongoing bias monitoring.
Employee Awareness Training
- AI tools should not simply be unleashed without appropriate guidance on how use the technology to get the most out of them… and how not to use them in order to avoid serious consequences
- Establish guidelines for using internal and external AI tools, and develop protocols for data handling in AI applications.
- Provide specialized training for AI developers and users.
- Create awareness campaigns on AI ethics and responsible use, particularly around using private or sensitive data.
- Build this training into your over-all security awareness programs, delivering messaging in a consistent, bite-sized, and engaging way.
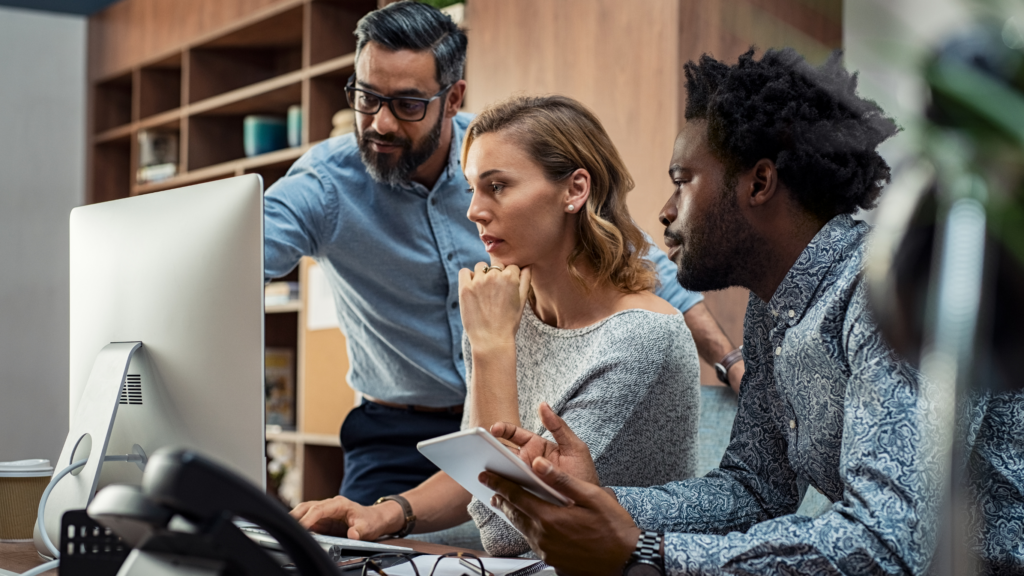
Step 6: Vendor Management
Most organizations have a mature process for vetting and managing their vendor partners. While it may be somewhat more challenging given the number and relative “newness” of players in the AI space, it’s still crucial to observe best practices:
Assessment and Oversight
- Develop criteria for evaluating AI vendors and tools, considering issues like experience, data residency, support, certifications, etc.
- Establish processes for vendor due diligence, and ongoing audit.
- Create guidelines for integrating external AI solutions.
- Implement monitoring systems for third-party AI services.
- Establish protocols for data sharing with external parties.
- Develop business continuity plans and contingency strategies to address vendor-related issues.
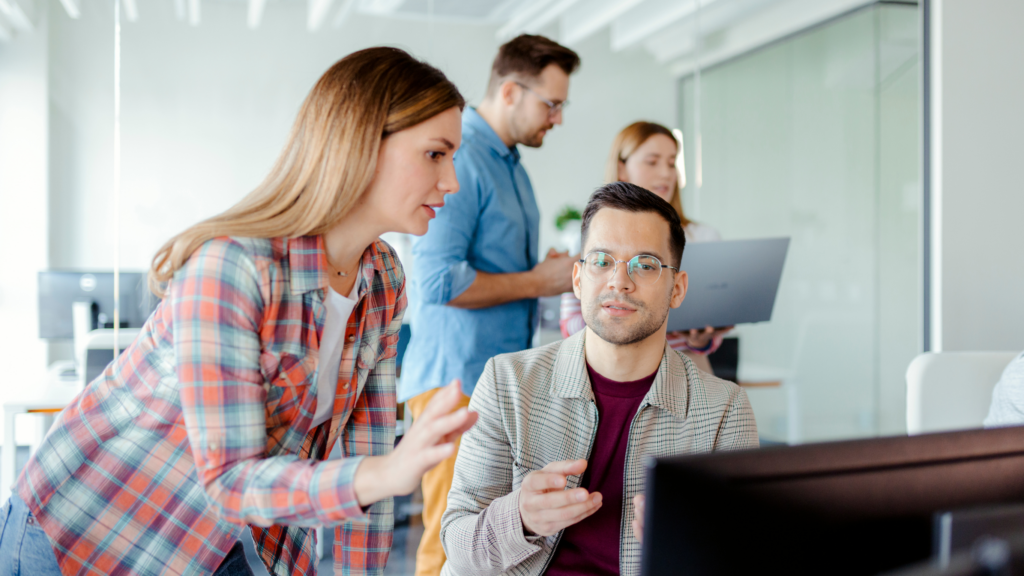
Step 7: Continuous Improvement and Feedback
Just as the AI space is fast-changing, your AI models will be evolving and changing from moment to moment as well. Performance monitoring and continuous refinement is vital, otherwise your policy and strategy will become out of date remarkably fast. Some key considerations to ensure that you keep pace:
Performance Monitoring
- Implement systems for ongoing AI performance evaluation.
- Establish key performance indicators (KPIs) for AI governance.
- Develop processes for continuous improvement of AI systems.
User Input
- Create channels for stakeholder feedback on AI systems.
- Establish processes for addressing AI-related concerns.
- Implement regular reviews of AI governance practices.
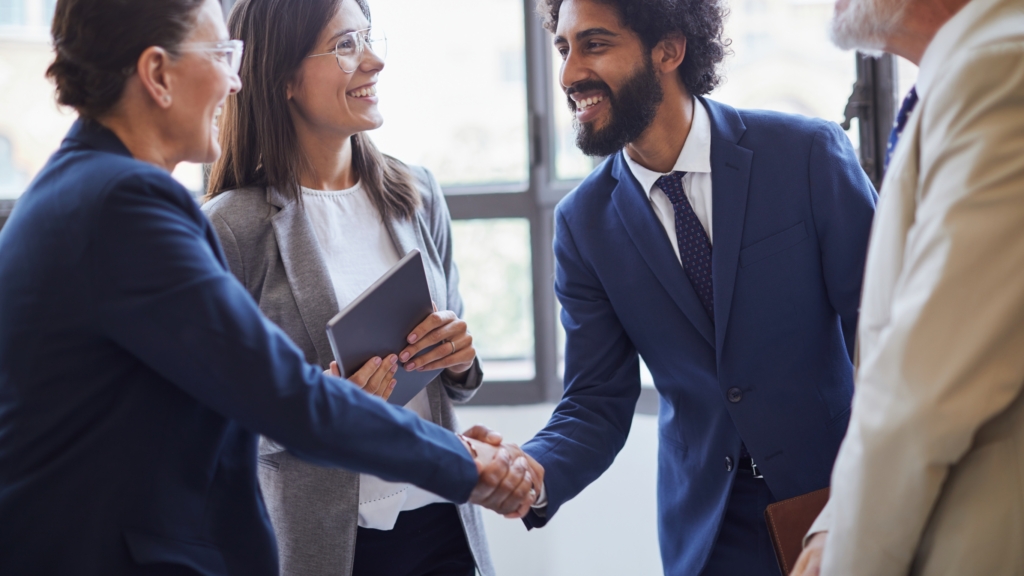
Step 8. Documentation and Reporting
Illustrating your good governance and compliance practices often comes down to documentation and metrics. Ensure that you have considered these areas during deployment – it can be a painful and time-consuming process to produce artifacts after the fact: look to have these elements created as an output of your normal operations, instead of a manual exercise at audit time.
Audit Trails
- Implement comprehensive logging of AI activities.
- Establish processes for maintaining audit trails.
- Develop protocols for internal and external audits.
Reporting
- Create regular reporting mechanisms on AI governance.
- Establish dashboards for AI performance and compliance producing performance-, risk- and compliance-based metrics.
- Develop processes for incident reporting and resolution.
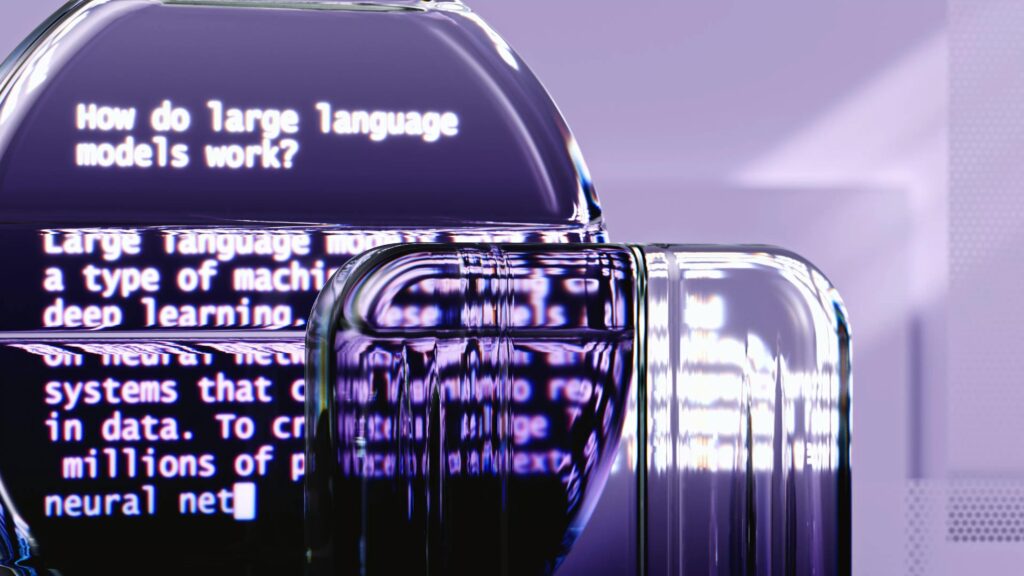
Helping you get started
While this just scratches the surface, this playbook will help guide you to create a comprehensive AI governance strategy that addresses key aspects of responsible AI use, data protection, and compliance. The process can be overwhelming: whether you’re just beginning your AI journey or looking to refine existing practices, we are here to help. Contact us today to learn more about how ISA Cybersecurity has implemented our own AI data governance strategy, and helped organizations like yours do the same.