Artificial intelligence (AI) and machine learning (ML) aren’t just buzz words anymore – they are key components of today’s cybersecurity landscape. Why? The rate of change in technology, the volumes of data involved, and sophistication of attackers – coupled with the well-documented global shortage of cybersecurity talent – have made it essential to leverage modern technologies to keep pace.
But many companies struggle to understand how to best leverage these advancements to enhance their security posture as there are so many use cases for AI and ML today.
To help companies get started or ramp up their adoption, this article will focus on three areas with the greatest impact:
1. Malware detection
2. Behavioural threat detection
3. Incident triage and response
1. Malware Detection
The days of simply using virus signatures to detect threats are long gone.
AI and ML techniques are required to detect and identify the limitless variety of cyber threats in the wild, whether they come from malware variants or variations on phishing emails. Current threat detection systems are able to defend against both known and unknown threats by using heuristic approaches that help systems learn on the fly.
Security platforms have evolved to sift through masses of data, looking for patterns of behaviour and isolating correlations at a speed and efficiency that cannot be matched by humans. Anomalies in real-time activities that might seem innocuous in isolation, suddenly come into focus when connected with other events on the network infrastructure. Modern malware detection solutions can detect, respond, and remediate – all in real-time.
2. Behavioural Threat Detection
Approaches like User and Event Behavioral Analytics (UEBA) use machine learning techniques to analyze and recognize typical behaviours and patterns in user accounts and endpoints, as well as identifying anomalous behavior that might signal an attack. UEBA can detect security incidents that violate predefined operational rules, employ novel attack patterns, or span multiple organizational systems and data sources. By using a “semi-supervised learning” approach – with humans providing the context while machines process data at high speed – threat detection and other defensive techniques can be developed that make sense of these massive streams and pools of information.
AL and ML techniques are being used extensively to develop threat intelligence to defend OT and IoT systems as well. Sectors like manufacturing, energy, and healthcare sectors – just to name a few – are supported by a myriad of smart devices. Signal processing analysis can help isolate exceptions in the masses of data created by operation and communication with these devices – exceptions that human observers or traditional detection methods simply cannot identify in real-time.
All of this machine-supported analysis helps identify isolate previously-unknown indicators of attack (IOAs) and indicators of compromise (IOCs), giving companies protection against zero-day threats or emerging internal threats that fall outside the parameters of day-to-day operations. Recognizing threats early gives organizations enough breathing room to block communications, adjust permissions, or safely apply patches in their environments.
3. Incident Triage and Response
AI and ML techniques are being used to streamline incident triage and response at the monitoring level as well. Machine learning is at its most powerful when faced with masses of data and precedent information, from which it can “learn” and provide analysis based on the past. In their managed security practice, ISA Cybersecurity has developed proprietary machine learning engines that filter noise, reduce false positives, and prioritize alerts – all of which helps improve the mean-time-to-detect (MTTD) threats against customers in ISA’s Cybersecurity Intelligence & Operations Centre (CIOC).
Rather than specifying the rules that require something to be flagged as a threat, machine learning engines can be shown examples of threats that have been detected in the past, and then use the machine learning to figure out what the rules should be, in order to flag those types of offenses. Semi-supervised learning is used to combine the best of the living and digital worlds.
Automation helps free up staff to focus on higher-value, actionable incidents without being distracted or burned out by investigating routine alerts. By employing contextual data supported by precedents, historical data, and correlation with threat intelligence feeds, technology can help teams gain a faster and more insightful handle on emerging incidents, reducing risk, and containing impact. And ISA Cybersecurity has seen that, when combined with SOAR capabilities, AI and ML has also driven down the mean-time-to-respond (MTTR) by deploying automated playbooks into action to quickly respond to threats. Automation finds commonalities across investigations and provides actionable feedback to analysts, freeing them up to focus on more important elements of the investigation and increase analyst efficiency.
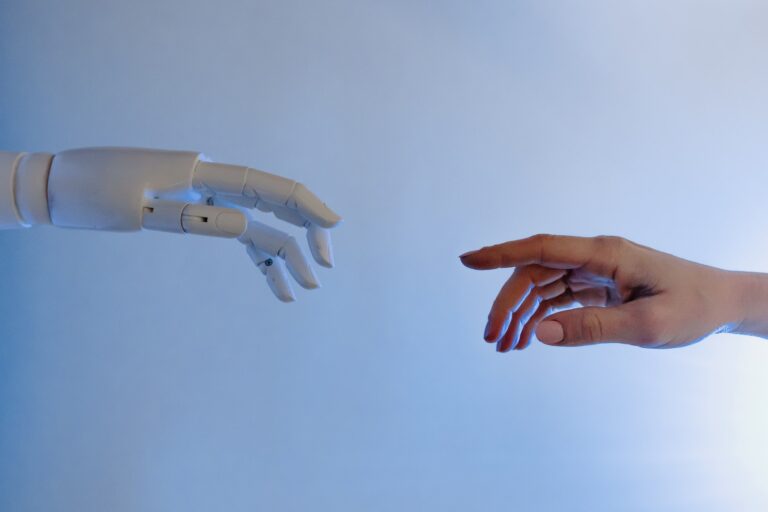
AI and ML on Offense
What’s another key reason AI and ML are so important in the cybersecurity world?
Some sophisticated threat actors are starting to use automation to boost their attacks too. Some ransomware-as-a-service and other hacking toolkits are using AI/ML techniques that can detect and avoid protections in place. Upon gaining a foothold in an unprepared system, hackers can move quickly and exploit opportunities for deeper infiltration by stealthily scanning for vulnerabilities at lightning speed. Modern malware can lie in wait, learning about the environment and devising evasion strategies, or even morph and re-write itself to avoid detection, based on what it has learned from defensive behaviours and actions it sees during operation.
Hackers are also using AI as a tool to trick defenses into thinking that threat activities are normal when they are not. AI can assist in creating “deep fakes” – realistic simulations of a user’s voice or image – in an effort to subvert authentication or spoof identity. Using a technique called “adversarial machine learning”, threat actors attempt to confound AI defenses by corrupting machine learning engines, purposely designing attack streams to misdirect AI decision processes, or even turning AI against itself through “model inversion attacks” that cause systems to reveal sensitive information from reconstructed AI training data from learning model parameters.
Indeed, using AI and ML in defensive strategies is a must-have, rather than a nice-to-have. With threat actors changing their tactics, and the underlying infrastructure that you’re trying to protect also changing – people are deploying new systems, onboarding new employees, all kinds of changes are happening in the areas you want to monitor – AI and ML techniques are essential to keep pace.
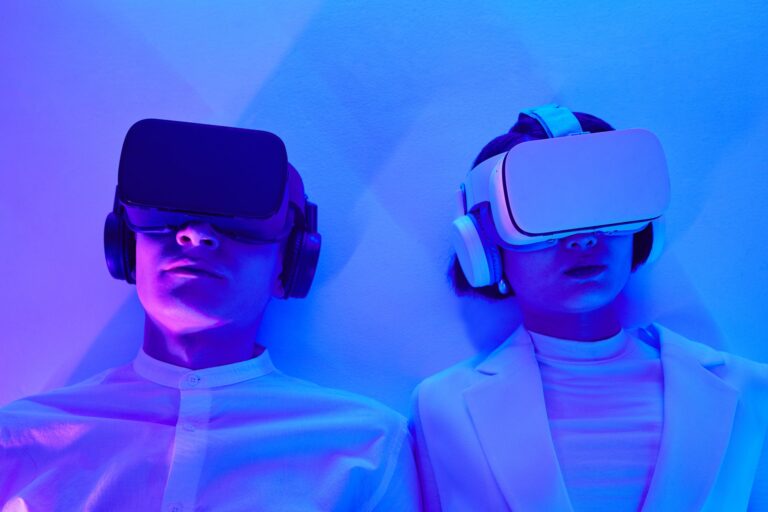
Other Applications of AI and ML in Cybersecurity
Research into improving defenses continues in the field every day. One of the more interesting fields involves natural language processing (NLP). NLP is used to teach systems to ingest and evaluate disparate sources of information, and distill that data into condensed analyses, insights, and reporting. ISA Cybersecurity has even turned to this technique to accelerate its own internal processes, using machine learning and fast-track service requests and document production. Every dollar saved on internal efficiencies is a dollar that can be invested in providing even better services for ISA Cybersecurity’s customers.
But AI and ML aren’t expected to completely replace people any time soon.The idea that machine learning is going to come in and magically get rid of all of your analysts and replace them with a machine learning algorithm is more of a fantasy than a reality. Machine learning is fast, but lacks certain things like being able to think creatively like humans can. The real secret sauce lies in being able to combine people with the machine learning algorithms for the best balance of speed and imagination.
Start leveraging AI and ML with Managed Services today
If you’d like to learn more about leveraging AI and ML to provide you with superior cybersecurity defenses, contact us today. You can enjoy cutting-edge technology through our managed services offerings, or partner with our professional services and consulting teams to discover the best ways to use these innovations yourself.